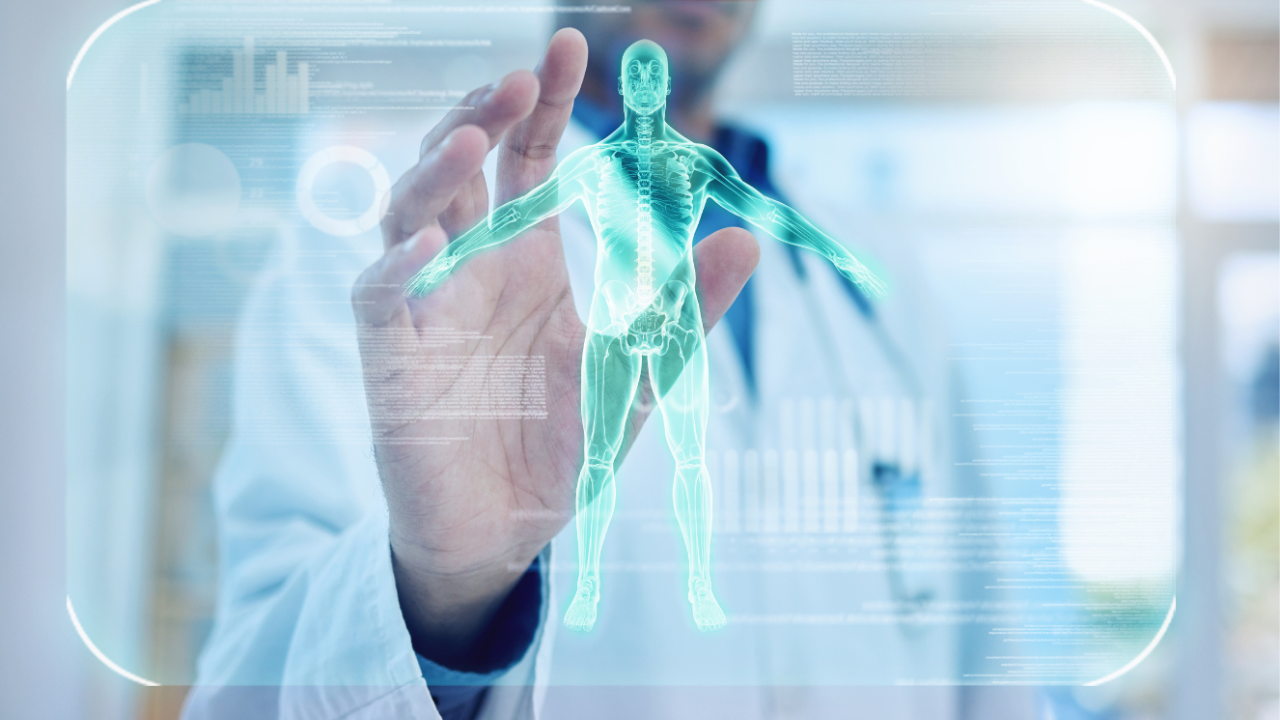
Mimicking the Minds of M.D.s
Artificial intelligence (AI) is poised to revolutionize healthcare, assisting doctors in diagnosing diseases, analyzing test results and predicting health risks. However, most AI systems focus on just one type of data at a time, limiting their ability to provide a comprehensive medical assessment. Unlike AI, doctors consider multiple factors—symptoms, medical history, lab results, and imaging scans—when making a diagnosis, leading to more accurate and informed decisions.
Geoffrey Tison, M.D., MPH, a cardiologist and researcher, is working to bridge this gap. With support from the UC Noyce Initiative, his team is developing an AI system that aims to better mimic the way doctors think by integrating different types of patient data simultaneously. The hope is that simultaneously considering multiple medical tests will improve the AI model to recognize patterns that a single test might miss, providing more complete insights into a patient’s health.
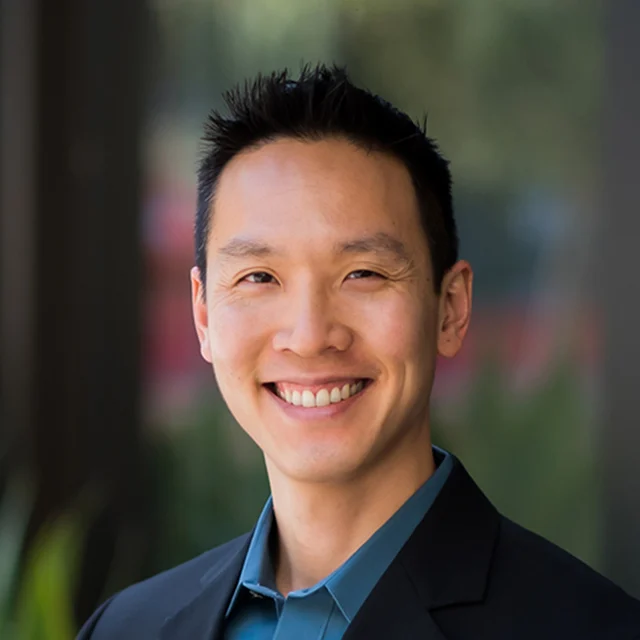
“Our goal is to provide AI with a greater breadth of data to allow it to think more like a doctor," Tison explained. “Instead of looking at just one piece of information, we want it to bring together different kinds of patient data—like blood tests and heart scans—to hopefully make a more accurate diagnosis.”
A Smarter AI for Detecting Heart Disease
To test this approach, the research team is focusing on a common but serious heart condition called Heart Failure with Reduced Ejection Fraction. This occurs when the heart isn’t pumping blood as well as it should, making it harder for the body to get the oxygen and nutrients it needs. The AI system they developed was able to analyze two different types of patient data at the same time—something most medical AI models can’t do. However, while the system successfully learned from both data sources, it didn’t yet outperform separate AI models trained on each data type individually.
How Transformers Make AI Smarter
At the heart of this innovation is a special kind of AI architecture called a transformer neural network. Originally designed for understanding human language (like the AI behind ChatGPT), transformers learn to focus on the most important parts of the data they’re analyzing. Tison and his team are using this technology to analyze medical data instead of text. The AI model learns which parts of a patient’s test results are most important and uses that information to make predictions about their health.
“Transformers are designed to figure out what really matters in a patient’s data,” Tison. “It’s similar to a doctor knowing which test results to pay close attention to and which ones aren’t as important.”
Applications to Other Health Issues
Developing a completely new way to train medical AI is a bold and challenging goal. Instead of following the usual methods used to build AI for medicine, Tison’s team is trying to redesign the process from the ground up.
“If we’re successful, this approach could change how AI is used in medicine,” he said, “not just for heart disease, but for many other conditions as well.”
UC Noyce Initiative has played a critical role in making this research possible, providing funding to support the team’s work and train the next generation of scientists. They funding has allowed them to involve a Ph.D. student and a postdoctoral researcher, giving them hands-on experience in cutting-edge AI research.
"The UC Noyce award gave us the chance to do something truly innovative,” Tison said.
Securing NIH Funding
The early success of this project helped Tison’s team secure a prestigious NIH New Innovator Award, a highly competitive grant that provides $1.5 million in funding over five years.
“The support from the UC Noyce helped us lay the foundation for this work,” Tison explained. “That foundation was key to securing additional funding, which will allow us to push this research even further.”
Tison continued, “Our long-term vision is to create AI models that better assist doctors by thinking more like them. By making AI more aware of the context across a broad range of patient data, we can build smarter tools that may ultimately improve patient care.”